Perceptual video compression
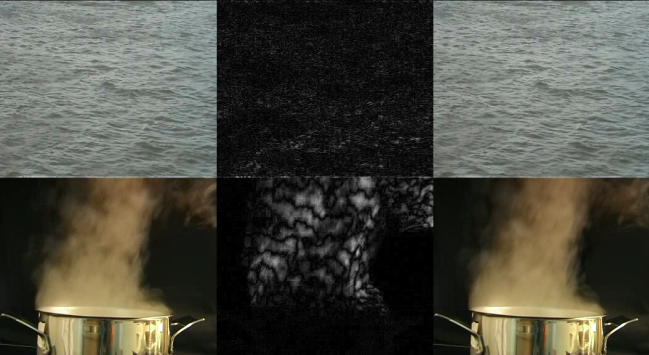
Visual experiences are key drivers, not just for the entertainment sector but also for business, security and communications technologies. CISCO predicts that video will soon account for over 80% of all internet traffic while mobile video access continues to rise by 100% year on year. These factors place increased demands on both communication networks and video compression.
The target of video compression is to provide good subjective quality rather than to simply produce the most similar pictures to the originals. Based on this assumption BVI researchers have conceived a compression scheme where an analysis/synthesis framework is employed rather than the conventional energy minimisation approach. This so-called parametric coding method employs a perspective motion model to warp static textures and utilises texture synthesis to create dynamic textures, similar to techniques used in computer graphics. The new algorithm offers significant potential for bitrate savings, compared to conventional methods. This work has spawned a major EU funded international collaboration (Provision) between Bristol, HHI Fraunhofer Berlin, The University of Nantes, The University of Aachen, BBC Research, Google YouTube, and Netflix to devise a future generation of video coding standards based on texture analysis and coding (David Bull, Aaron Zhang, Angeliki Katsenou, Mariana Afonso, Dimitris Agrafiotis, Miltiadis-Alexios Papadopoulos, Thomas Ntasios).
One of the key challenges in video compression is how to measure the perceptual quality of a distorted image or video. Visual perception is highly complex, influenced by many confounding factors, not fully understood and difficult to model. Because the perceptual distortion experienced by the human viewer cannot be fully characterised using simple mathematical differences, BVI researchers Aaron Zhang and David Bull have developed a new Perceptual Video Metric called PVM. PVM simulates perception processes by adaptively combining noticeable distortions with measures of typical artefacts such as blurring and offers better correlation with human opinions than any of its competitors.