Faculty of Science and Engineering
Study with us
Undergraduate
Find undergraduate courses.
Postgraduate taught
Find postgraduate taught programmes and courses.
Postgraduate research
Find PhD opportunities and funding.
Partners and outreach
Science Partnership Office
The Science Partnership Office (SPO) supports and promotes long-term partnerships between scientists, industry, government and the community.
Industrial Liaison Office
The Industrial Liaison Office (ILO) helps industry to engage with both students and academics in Engineering subjects.
Faculty outreach activities
We're passionate about giving school-aged children opportunities to create, explore and learn about the latest ideas in science, engineering, computing and mathematics.
Schools
News
Keep up to date with the latest faculty news.
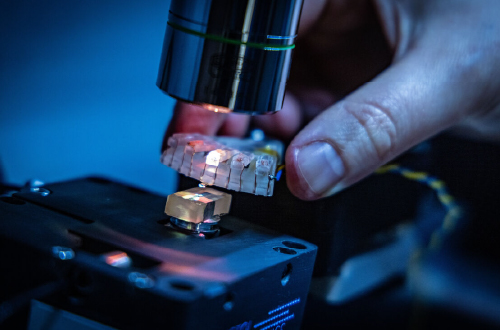
Contact us
Get in touch with us - faculty contacts and information.